AI
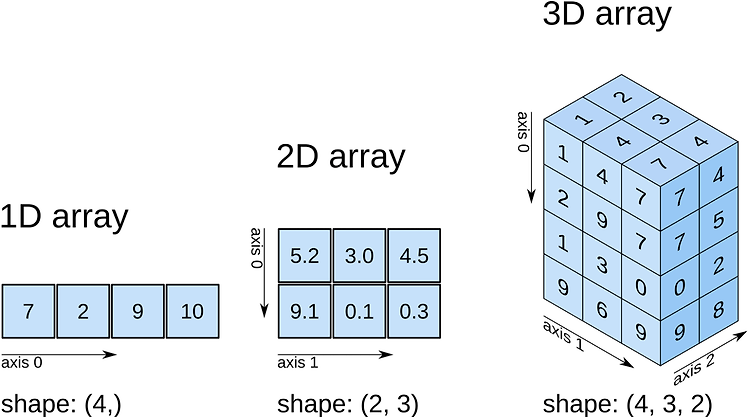
numpy.newaxis는 어떻게 작동하며 언제 사용합니까?
간단히 말해, numpy.newaxis는 numpy array의 차원을 늘려준다고 보면 된다. 1D 배열은 2D 배열이 됩니다 2D 배열은 3D 배열이 됩니다 3D 배열은 4D 배열이 됩니다 4D 배열은 5D 배열이 됩니다 다음은 1D array에서 2D array로 차원을 늘려주는 그림이다. np.newaxis를 사용함에 있어 3가지 정도의 시나리오가 적합해 보인다. 시나리오-1 1D array를 row vector나 column vector로 사용하고 싶을 경우 numpy에서 array를 만들면 shape은 아래와 같이 나타낸다. 1 2 3 4 # 1D array In [7]: arr = np.arange(4) In [8]: arr.shape Out[8]: (4,) Colored by Color Sc..
![[Application & Tips] Efficient Models](https://img1.daumcdn.net/thumb/R750x0/?scode=mtistory2&fname=https%3A%2F%2Fblog.kakaocdn.net%2Fdn%2FBNIxC%2Fbtq6Z9iKJeE%2F4kKeGRIwAVb8TB1AOOYQN1%2Fimg.png)
[Application & Tips] Efficient Models
[TensorFlow] Lab-07-3-1 application and tips 모두의 딥러닝 시즌 2 정리... 시즌2 강의가 부실하다고 판단되어 시즌 1 lec 07-2: Training/Testing 데이타 셋 강의 내용 추가 정리 목차 Data sets Evaluation using training set? Training and Test sets Trainig / Validation / Testing Learning Online Learning vs Batch Learning Fine tuning Efficient Models Sample Data MNIST / Fashion MNIST / IMDB / CIFAR-100 Efficient Models 실제 우리가 fine-tuning이라던지 여러..
![[Application & Tips] 학습 전략 - Transfer Learning / Fine Tuning / Feature Extraction](https://img1.daumcdn.net/thumb/R750x0/?scode=mtistory2&fname=https%3A%2F%2Fblog.kakaocdn.net%2Fdn%2Fvu8dK%2Fbtq60lDetAw%2Fpvpk9humeksWO2w40apxqK%2Fimg.png)
[Application & Tips] 학습 전략 - Transfer Learning / Fine Tuning / Feature Extraction
[TensorFlow] Lab-07-3-1 application and tips 모두의 딥러닝 시즌 2 정리... 시즌2 강의가 부실하다고 판단되어 시즌 1 lec 07-2: Training/Testing 데이타 셋 강의 내용 추가 정리 목차 Data sets Evaluation using training set? Training and Test sets Trainig / Validation / Testing Learning Online Learning vs Batch Learning Fine tuning Efficient Models Sample Data Fashion MNIST / IMDB / CIFAR-100 Transfer Learning / Fine Tuning / Feature Extracti..
![[Application & Tips] Online Learning vs Batch Learning](https://img1.daumcdn.net/thumb/R750x0/?scode=mtistory2&fname=https%3A%2F%2Fblog.kakaocdn.net%2Fdn%2FFSNUU%2Fbtq6W1scK6o%2Fi700uBRQUrUKjn2SoSurHK%2Fimg.png)
[Application & Tips] Online Learning vs Batch Learning
[TensorFlow] Lab-07-3-1 application and tips 모두의 딥러닝 시즌 2 정리... 시즌2 강의가 부실하다고 판단되어 시즌 1 lec 07-2: Training/Testing 데이타 셋 강의 내용 추가 정리 목차 Data sets Evaluation using training set? Training and Test sets Trainig / Validation / Testing Learning Online Learning vs Batch Learning Transfer Learning / Fine Tuning / Feature Extraction Efficient Models Sample Data Fashion MNIST / IMDB / CIFAR-100 Online vs..
![[Application & Tips] Data sets & Learning](https://img1.daumcdn.net/thumb/R750x0/?scode=mtistory2&fname=https%3A%2F%2Fblog.kakaocdn.net%2Fdn%2FZUstd%2Fbtq4t4ecO68%2FjhzPWw2WXWeVUHODg7fZA1%2Fimg.png)
[Application & Tips] Data sets & Learning
[TensorFlow] Lab-07-3-1 application and tips 모두의 딥러닝 시즌 2 정리... 시즌2 강의가 부실하다고 판단되어 시즌 1 lec 07-2: Training/Testing 데이타 셋 강의 내용 추가 정리 목차 Data sets Evaluation using training set? Training and Test sets Trainig / Validation / Testing Learning Online Learning vs Batch Learning Fine tuning Efficient Models Sample Data Fashion MNIST / IMDB / CIFAR-100 Performance Evaluation : is this good? 지금까지 우리는 배운..
![[Application & Tips] Overfitting](https://img1.daumcdn.net/thumb/R750x0/?scode=mtistory2&fname=https%3A%2F%2Fblog.kakaocdn.net%2Fdn%2Fcw36vp%2Fbtq4eiYxUQX%2FglqFX1vlUKQ5JKBsFpl4JK%2Fimg.png)
[Application & Tips] Overfitting
[TensorFlow] Lab-05-2 Logistic Regression 모두의 딥러닝 시즌 2 정리... 목차 Learning rate Gradient Good and Bad Learning rate Learning rate schedule 과 Annealing the learning rate (Decay) Data preprocessing Feature Scaling - Standardization / Normanalization Noisy Data Overfitting Set a Feature Regularization overfitting에 관해 설명하기에 앞서 그래프를 통해 간단하게 설명하고 지나가겠다. 위의 그래프를 살펴보면 실제 우리가 모델을 만드는 과정에서는 Validation data ..
![[Application & Tips] Data preprocessing](https://img1.daumcdn.net/thumb/R750x0/?scode=mtistory2&fname=https%3A%2F%2Fblog.kakaocdn.net%2Fdn%2FqlY6m%2Fbtq4jsrXSAK%2FKFTeDIsLTLAtECSAUO0oik%2Fimg.png)
[Application & Tips] Data preprocessing
[TensorFlow] Lab-05-2 Logistic Regression 모두의 딥러닝 시즌 2 정리... 목차 Learning rate Gradient Good and Bad Learning rate Learning rate schedule 과 Annealing the learning rate (Decay) Data preprocessing Feature Scaling - Standardization / Normanalization Noisy Data Overfitting Regularization L2 Norm 이전 글에서 Learning rate를 이용한 학습 응용을 살펴보았다. 이젠 AI에서 제일 중요한 데이터 전처리에 대해 설명하고자 한다. 데이터 전처리는 머신러닝 과정의 70~80%를 차지하..
![[Application & Tips] Learning rate](https://img1.daumcdn.net/thumb/R750x0/?scode=mtistory2&fname=https%3A%2F%2Fblog.kakaocdn.net%2Fdn%2Fbdqs93%2Fbtq37zyx5Ux%2F2HLWs2YC40HIetMsinwJJ1%2Fimg.png)
[Application & Tips] Learning rate
[TensorFlow] Lab-05-2 Logistic Regression 모두의 딥러닝 시즌 2 정리... 이번 포스팅부터는 지금까지 배운 이론들의 응용 방법과 tip에 관한 내용들이다. 강의에서 말하길 지금까지 배운 이론들은 실제 실무에선 많은 차이와 적용의 어려움이 있다고한다. 그래서 [Application & Tips] 강의를 통해 이러한 차이를 줄이길 바라면서 정리를 해보겠다. 목차 Learning rate Gradient Good and Bad Learning rate Learning rate schedule 과 Annealing the learning rate (Decay) Data preprocessing Standardization / Normanalization Noisy Data Ov..
![[Softmax Regression] Softmax classifier 의 cost함수](https://img1.daumcdn.net/thumb/R750x0/?scode=mtistory2&fname=https%3A%2F%2Fblog.kakaocdn.net%2Fdn%2FuuEQB%2Fbtq3v7PPWzY%2Funx7AvNTraf9htjm97zIq1%2Fimg.png)
[Softmax Regression] Softmax classifier 의 cost함수
[TensorFlow] Lab-05-2 Logistic Regression 모두의 딥러닝 시즌 2 정리... 복습... 지난 포스팅에서 우리는 오른쪽의 도식화된 것처럼 각각의 classifier을 이용해 계산하는 것보다 하나의 큰 Weight matrix를 갖고 계산하는 것이 더 편리하고 각각의 classifier의 결과 값을 갖는 하나의 벡터로 결과가 나오게 된다고 하였다. 이를 간단하게 도식화해보면 다음과 같다. 이때 \(Y_{A}\), \(Y_{B}\), \(Y_{C}\) 각각에 해당하는 실수 값들로 결과가 반환되었다. 하지만 우리가 원하는 바는 실수 값인 결과가 아닌 sigmoid 같은 것을 사용하여 0~1 사이의 값들로 반환되기를 원하였다. 이를 그림을 통해 간단하게 정리하면서 다음과 같다. S..
![[Softmax Regression] Multinomial classification](https://img1.daumcdn.net/thumb/R750x0/?scode=mtistory2&fname=https%3A%2F%2Fblog.kakaocdn.net%2Fdn%2Fcah14I%2Fbtq3nIxggT8%2FGZ6w8eDf170aeEFtRWGtn1%2Fimg.png)
[Softmax Regression] Multinomial classification
[TensorFlow] Lab-05-2 Logistic Regression 모두의 딥러닝 시즌 2 정리... 지난 포스팅에서 Logistic Regression에 대해 정리를 하였다. 이에 대해 간단하게 복습을 해보자면... 우리는 Logistic regression 중 binary 결과 값에 대한 예측을 진행하였다. 0 or 1로만 구분하는 것!! 초기에 우리의 H(x) = Wx와 같은 linear 한 hypothesis에서 출발하였다. 그런데 이런 H(x) = Wx 의 단점은 리턴하는 값이 실수 값이기 때문에 0, 1과 같은 binary 한 결과를 고르는 것에는 적합하지 않았다. 그래서 우리는 g(H(x))을 통해 실수 값으로 크게 분포되어 있던 값들을 0~1 사이의 값으로 압축하는 과정을 진행하였다..